2023 Blog, Research Gateway, Blog, Command blog, Feature Blog, Featured
Secure Research Environments provide researchers with timely and secure access to sensitive research data, computation systems, and common analytics tools for speeding up Scientific Research in the cloud. Researchers are given access to approved data, enabling them to collaborate, analyze data, share results within proper controls and audit trails. Research Gateway provides this secure data platform with the analytical and orchestration tools to support researchers in conducting their work. Their results can then be exported safely, with proper workflows for submission reviews and approvals.
The Secure Research Environments build on the original concept of Trusted Research Environment defined by UK NHS and uses the five safes framework for safe use of secure data. The five elements of the framework are:
- Safe people
- Safe projects
- Safe settings
- Safe data
- Safe outputs
There are the following key building blocks for the solution:
- Data Ingress/Egress
- Researcher Workflows & Collaborations with costs controls
- On-going Researcher Tools Updates
- Software Patching & Security Upgrades
- Healthcare (or other sensitive) Data Compliances
- Security Monitoring, Audit Trail, Budget Controls, User Access & Management
The figure below shows implementation of Secure Research Environments solution with Research Gateway.
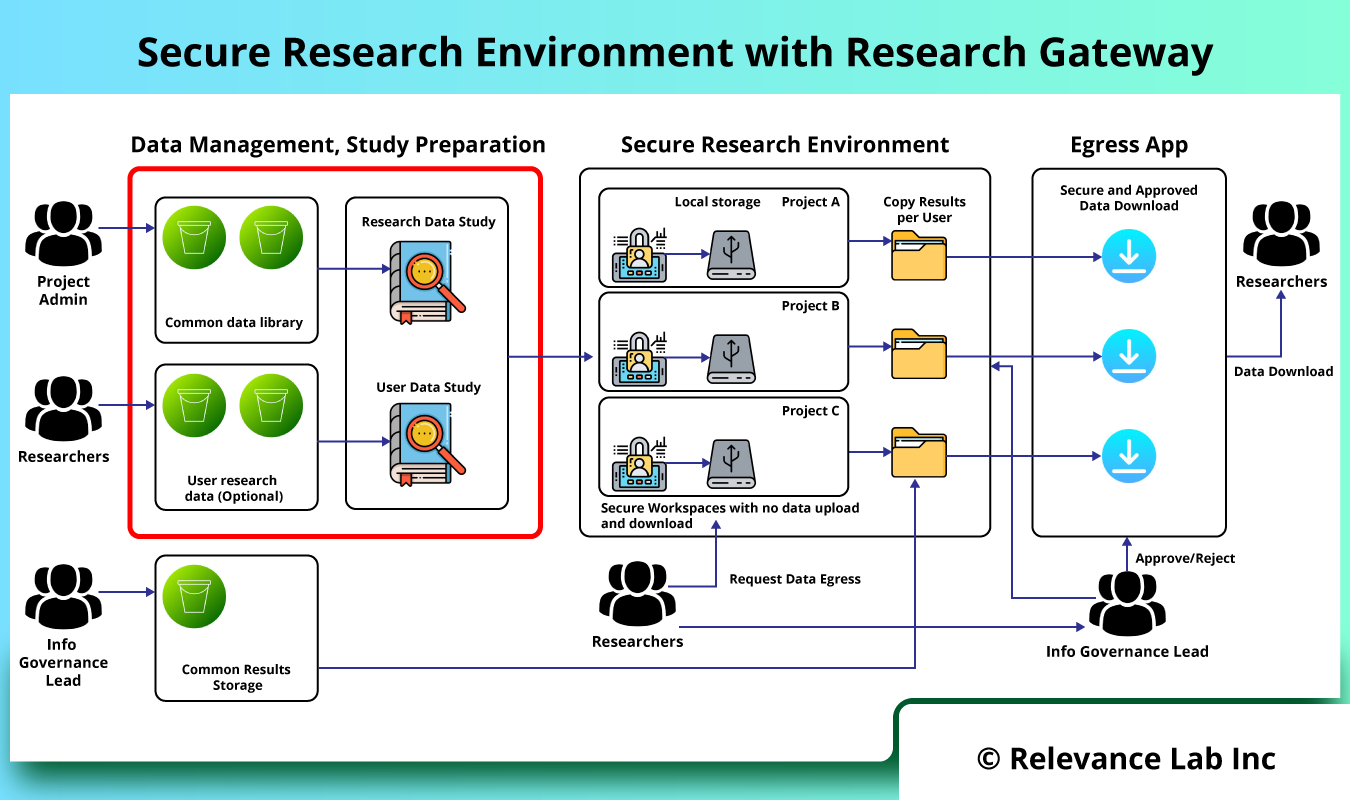
The basic concept is to design a secure data enclave in which there is no ability to transfer data into or out of without going through pre-defined workflows. Within the enclave itself any amount or type of storage/compute/tools can be provisioned to fit the researcher’s needs. There is capability to use common research data and also bring in specific data by researchers.
The core functionality for Secure Research Environments deal with solutions for the following:
Data Management and Preparation
This deals with “data ingress management” from both public and private sources for research. There are functionalities dealing with data ingestion, extraction, processing, cleansing, and data catalogs.
Study Preparation
Depending on the type of study and participants from different institutions, secure data enclave allows for study specific data preparation, allocation, access management and assignment to specific projects.
Secure Research Environment
A controlled cloud environment is provided for researchers to access the study data in a secure manner with no direct ingress-egress capability and conduct research using common tools like JupyterLab, RStudio, VSCode etc. for both interactive and batch processing. The shared study data is pre-mounted on research workspaces making it easy for researchers to focus on analysis without getting into complexity of infrastructure, tools and costs.
Secure Egress Approvals for Results Sharing
Post research if researchers want to extract results from the secure research environment, a specialized workflow is provided for request, review, approvals, and download of data with compliance and audit trails.
The Secure Research Environments Architecture provides for Secure Ingress and Egress controls as explained in the figure below.
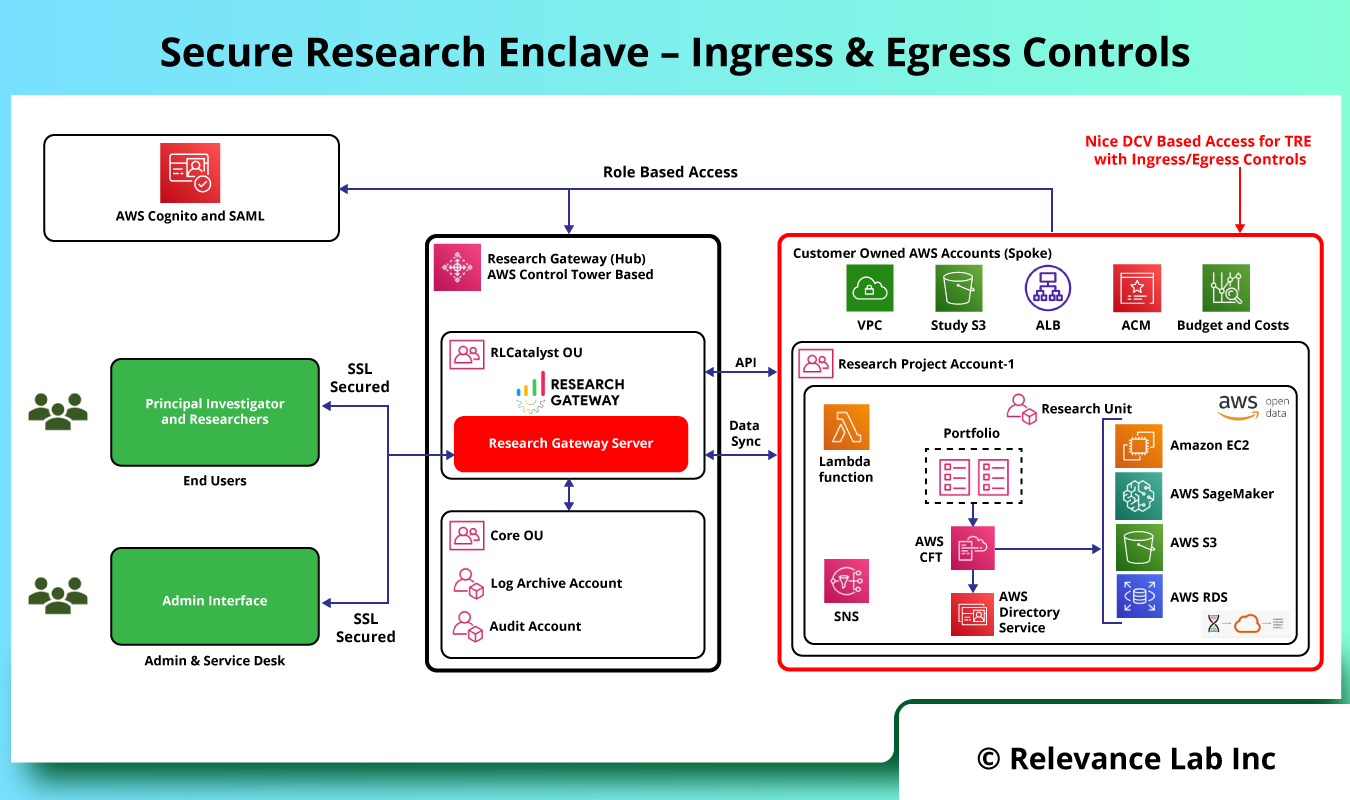
Building Block | Detailed Steps |
Data Management |
|
Secure Research Environments |
|
Egress Application |
|
The need for Secure Research Enclave is a growing one across different countries. There is an emerging need for a consortium model, where multiple Data Producers and Consumers need to interact in a Secure Research Marketplace Model. The marketplace model is implemented on AWS Cloud and provides for tracking of costs and billing for all participants. The solution can be hosted by a third-party and provide Software as a Service (SaaS) model driving the key workflows for Data Producers and Data Consumers as explained in figure below.
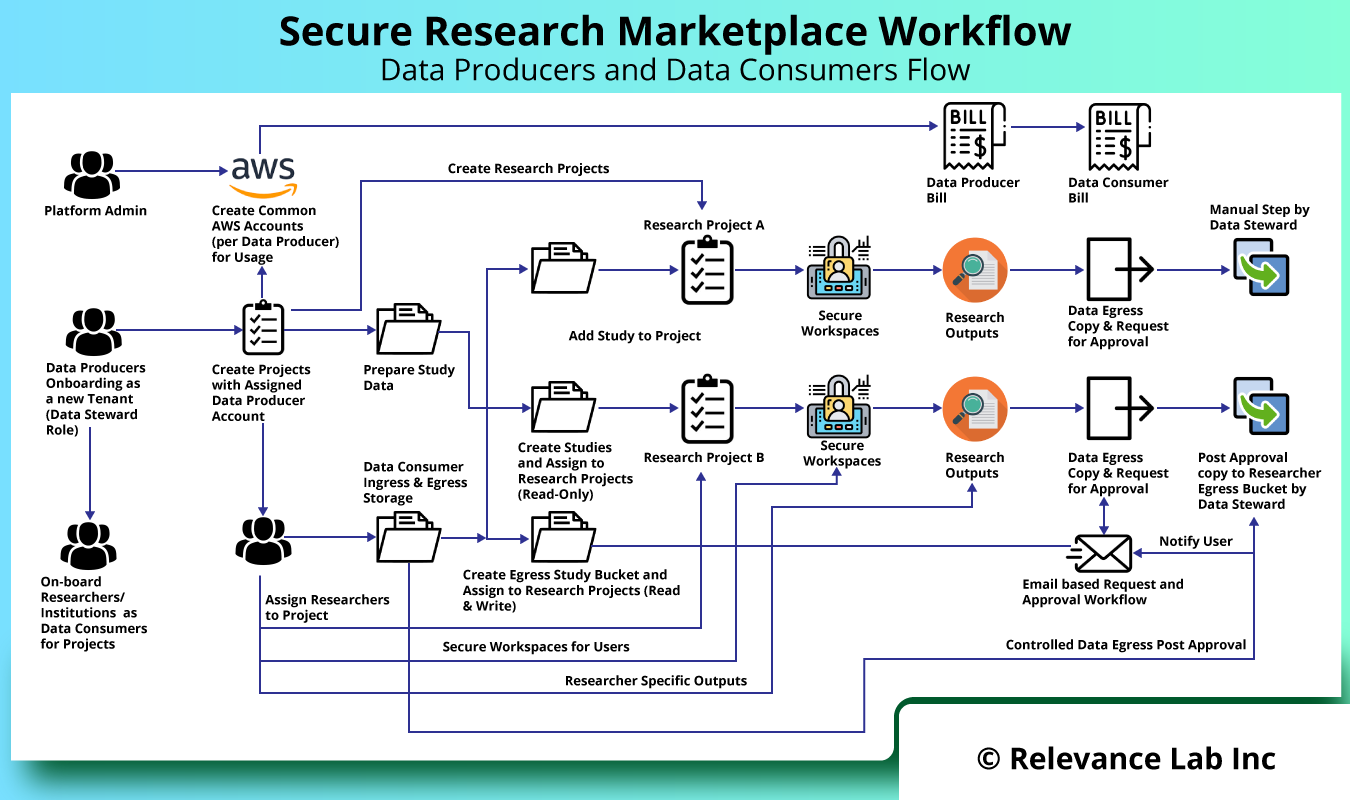
Summary
Secure Research Environments are key features for enabling large institutions and governmental agencies to speed up research across different stakeholders leveraging the cloud. Relevance Lab provides a pre-built solution that can speed up the implementation of this large scale and complex deployment in a fast, secure, and cost-effective manner.
Here is a video demonstrating the solution.
To know more about this solution, feel free to write to marketing@relevancelab.com.
References
UK Health Data Research Alliance – Aligning approach to Trusted Research Environments
Trusted (and Productive) Research Environments for Safe Research
Deployment of Secure Data Environments on AWS
Microsoft Azure TRE Solution