2019 Blogs, Blog
In last year’s Google I/O conference, when Sunder Pichai showed the demo of an AI assistant that can schedule appointments, make calls on our behalf, book tables at a restaurant etc, all the imaginations about AI came into reality. It felt as though Pichai was talking to the Genie of Aladdin who fulfils day-to-day mundane operational tasks that can be made simpler with the help of Artificial Intelligence. Similarly, the mission of AIOps is to make the job of IT Operations simpler and more efficient.
According to Gartner, AIOps—the “Artificial Intelligence” for IT Operations—is already making waves in the way IT Ops team work. One of the important use cases of digital technologies is how AIOps is becoming pervasive in the IT world and how it is transforming the traditional IT management techniques. While digital transformation enhances Cloud adoption for enterprises, there is an increasing need to manage “Day 2 Cloud scenario” more efficiently in order to realize the true benefits of cloud transformation. AIOps helps IT Operations teams automate and enhance their operations using analytics and machine learning. This enables them to analyze data collected from various tools and devices and predict and resolve IT issues in real-time, ensuring that business services are always available to business users. This is important for any organization that operates in a “service-driven” environment.
Here are various components of AIOps:
1) Data Ingestion: This is a core capability of any AIOps tool. These tools process data from disparate and heterogeneous sources. The principle of AIOps is based on the technique of machine learning and data crunching. Hence, it is important to ingest various datasets available that determine the key success parameters for IT operations. They involve data collected from various performance monitoring tools, service desk ticket data, individual system metrics, network data etc. Due to the voluminous and exponentially increasing nature of data, it is very difficult to track all these datasets manually and determine their impact on day-to-day IT Operations.
2) Forming the Model/Anomaly Detection: Once the data ingestion layer is created, the next important aspect of an AIOps system is the ability to form a model of what is normal. Once the system forms this, the capability of Anomaly detection can be built on that. Any parameter that deviates from normal can be flagged as an anomaly that could lead to outages, hampering the availability of business services. Machine learning can be applied for Anomaly detection. However, it is best applied to specific use cases where patterns and actions are repeatable. This is the step where self-learning capabilities are injected in the system.
3) Prediction of Outages: Once the system starts determining what is normal and what is an anomaly, it becomes easier to predict outages, performance degradation or any other condition that affects the overall business built on the model. For instance, an increase in database queue sizes could lead to increase in transaction times for online payments, which could in turn lead to abandoned items in shopping carts. The AIOps tool should be able to predict such a pattern and flag it.
4) Actionable Insights: The system should be able to look at past data of actions taken and provide recommendations on the possible actions that can prevent downtime of business services. Past actions could be tracked via the ITSM tickets created for past incidents or through knowledge-base articles that have been associated with those tickets.
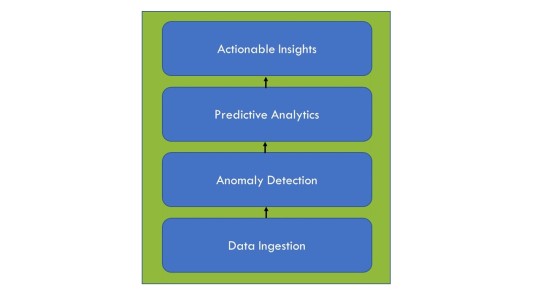
One of the important use cases for AIOps that we have been implementing for our enterprise client is storage management. In a typical production environment, IT Operations team would get an alert when the disk is close to its full capacity. As a result of this, the responses from a particular node become weaker. Through intelligent monitoring and correlation analysis, the exact reasoning can be determined and the storage capacity is automatically adjusted by adding new volumes proactively and functioning of that node can be restored to normal level.
There are other use cases of AIOps in capacity management, resource utilization etc. which could make the life of IT Ops team much simpler. The day is not far when a CIO takes the avatar of Aladdin and the Genie shows up in the form of an AIOps tool.
About the Author:
Sundeep Mallya is the Vice President and Head of Engineering for RL Catalyst Product at Relevance Lab.